Are your product engineering teams using AI? Our study reveals they should be.
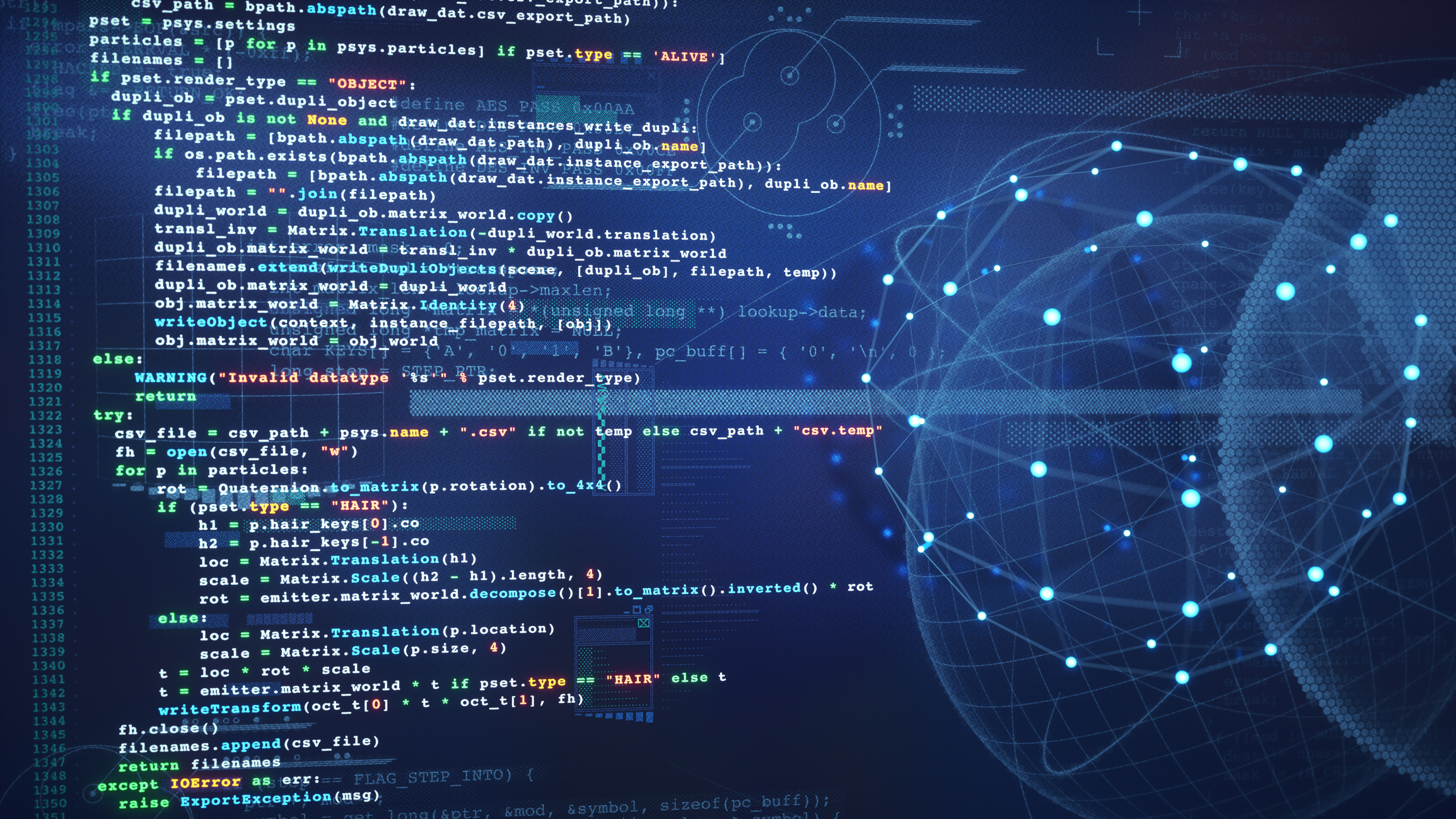
AI is no longer just a tool – it’s a teammate.
Major tech giants like OpenAI, GitHub, Microsoft and Google are pushing the boundaries of what AI can achieve in software engineering – and the reception has been a healthy mix of excitement and scepticism.
But we saw an unanswered question: How effective is AI in enhancing the quality and speed of product engineering?
This blog post delves into our research, exploring the profound impact AI tools like ChatGPT, GitHub, Copilot and IntelliJ AI Assistant have on product engineering.
Our goal? To quantify the potential of AI in augmenting problem-solving skills, but also to identify best practices and areas where AI may not be as effective.
By understanding these dynamics, we can understand how to better integrate AI tools into the day-to-day workflows of delivery teams, maximising productivity and improving quality.
And the results reveal a promising horizon for AI’s role in the field.
Our study, at a glance
We tested AI’s influence on product engineering in a detailed study involving 53 professionals ranging from software engineers to product managers.
The study spanned four crucial tasks: unit test writing, code conversion, feature building and modernising code.
To get a clear contrast in performance, participants tackled these tasks both with – and without – AI assistance.
We also followed an iterative approach – with two rounds of experiments. The second round was designed to incorporate learnings from the first. Specifically, to test whether or not additional time to allow for upskilling in the use of the tools provided would lead to better performance.
What did we find?
The overarching result was clear – AI tools significantly improved both the speed and quality of product engineering tasks. For instance, in unit testing, 70% of the participants saw a quality boost with AI assistance and 58% reported quicker completion in code conversions.
Let’s break it down task by task:
Unit testing
Headline findings:
- 65% of the participants completed the task faster with AI
- 70% achieved higher quality scores
What does this mean for product engineering?
This shows that AI can significantly accelerate task completion while enhancing output quality – it’s a win win. For engineering teams, this means reduced development cycles and improved code reliability, leading directly to faster product iterations overall.
Modernising code
Headline findings:
- 65% of participants improved both speed and quality with AI assistance
- The average quality improvement stood at 37%
What does this mean for product engineering?
The future is bright with AI showing it can hugely improve the modernisation of legacy systems, allowing teams to achieve more, in less time – but at a higher quality.
Feature building
Headline findings:
- Nearly half of study participants improved both the speed and quality of their work
- The average time saved increased dramatically in the second round to 40%
What does this mean for product engineering?
The use of AI in feature development not only speeds up the engineering process but also improves the end product. This capability supports rapid prototyping and testing, allowing teams to refine features quickly and meet tight launch timelines.
Code conversion
Headline findings:
- 73% of participants noted quicker task completion
- Quality improvements were consistent, peaking at 69% of participants
What does this mean for product engineering?
An AI and code conversion combination can save a bundle of time and while also boosting quality. This will allow teams to handle larger codebases more effectively, making sure upgrades and migrations are less resource-intensive and more reliable.
Final thoughts
The current results show that there’s a big opportunity to significantly improve both quality and speed – with the right training, experience and guidance.
This is based on the results and also the feedback collated throughout the experiments. Change can be scary and perception can make or break adoption. The truth is, the training effort is small in comparison to the speed and quality benefits.
For us, we believe that this is the boost that product engineering teams need to flourish in the future – and today.
And we haven’t stopped with just this research – AI is constantly evolving, so our understanding of how it fits into product engineering has to evolve too. Watch this space for more first-hand CreateFuture research on how product engineers can take AI, and run with it.
In the meantime, you can download our latest guide where we dive deeper into the key insights of our most recent study to unlock the tangible benefits of AI.